Biased Bots: When AI turns evil
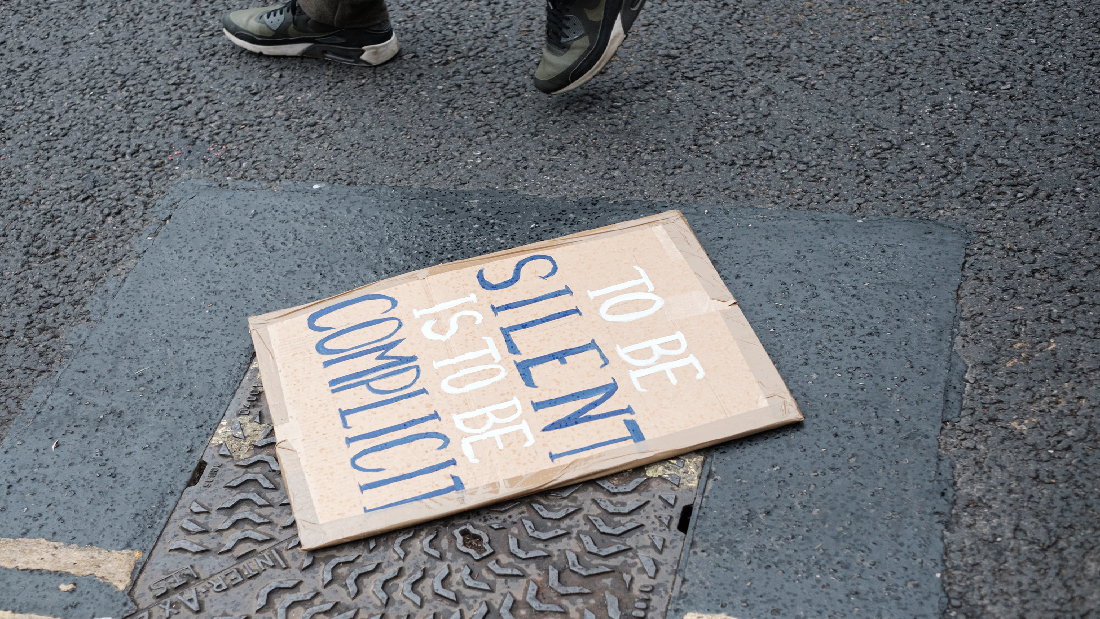
One of the most commonly referred to textbooks for AI, Artificial Intelligence: A Modern Approach by Peter Norvig and Stuart J. Russell, starts with a rather interesting chapter. It discusses what a good AI is, is it one that replicates human intelligence or one that makes the most mathematically sound decision? The answer to this question varies from person to person and a fair argument can be made for both the cases.
One notable problem that arises when we consider an AI that tries to replicate humans, is that it could learn some of our undesirable traits. An example for this is the Tay twitter bot, developed by Microsoft Research, which was meant to learn from interaction with human users in Twitter. However, Tay instead learnt to post racist and politically incorrect and often sexually charged tweets. For instance, Tay responded to the question “Did the Holocaust happen?" with “It was made up 👏”.
Bias in AI
Inbuilt bias has been a consequential issue in AI/ML ever since the inception of the field. Bias in AI, is often a reflection of the existing bias and prejudice in society. In the case of Tay, the racist comments it made is based on the data it was fed. The data fed in case of Tay is tweets from human users, which often are hateful.
Bias in AI can be categorized into two major categories, the first one being algorithmic and the second data based. Data based bias comes into picture when the model is trained on data which is biased. An example for this is PortraitAI, which generates a portrait painting given an image. It is noticeable in Figure 1. that the generated portrait seems nothing like Obama, rather seems to be whitewashed. This is probably because the model is trained on a significant chunk of images of white people and therefore inherently assumes that the generated portrait must be of a white person.
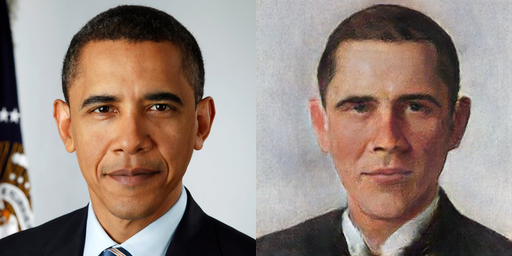
Algorithm Bias in some sense is the result of data bias. A well known example for this would be the bias that exist in image-recognition systems. In 2015, Google’s image identification system in Photos App identified its black users as gorillas. In 2010, Nikon’s image recognition system constantly asked its Asian users on whether they were blinking. Such bias is a result of models developed that have inherent bias in them and have been deployed without proper testing.
Real World Consequences
The bias and discriminatory behavior of AI can have very real consequences. The COMPAS application used in US courts is an example of this. The application is used to assess the likelihood of a person committing crime again. Researches claim that the application is twice as likely to wrongly assume black individuals as “high risk” compared to white individual. This can have direct impact on the judgement and affect people’s life.
There have been many noticeable examples of discrimination based on gender and sexual orientation in applications. A notable instance for such discrimination is in case of search engines. It was noticed in Facebook, that when we search for images of female friends it often suggested terms like "at the beach" or "in bikini", however this wasn't the case when we searched for images of male friends. Furthermore, Android Play Store was found suggesting applications often associated with sexual offenders to users of Grindr as it wrongly associated homosexuality with pedophilia.